Vice President, Audiology &
Professional Relations
Oticon, Inc.
Introduction
I visited my favorite internet news site this morning and found a story about a new "smart" running shoe. The shoe has a computer chip on-board that senses the runner's size and stride length and then directs on-going changes in the heal cushioning via a miniature screw and cable system. According to a spokesman for Adidas, the shoe "senses, understands and adapts" (McCall, 2004).
Artificial Intelligence, the ability of computers to use advanced problem solving approaches to complex situations, is all around us. It's used in everyday consumer items such as robotic vacuum cleaners and running shoes, all the way up to advanced aeronautic navigation systems and medical computer imaging systems.
What is Artificial Intelligence?
Artificial Intelligence (AI) in general, describes a field of computer science in which programs are created to apply multi-dimensional, intelligent solutions to complex problems. One of the most common applications is to process large amounts of information and, by either following or deducing rules, determine an appropriate response to this input.
When considering AI, many people think of the science fiction version of intelligent, interactive robots ranging from "R2D2" and "C3P0" of the Star Wars series or "HAL" from 2001: A Space Odyssey. However, Artificial Intelligence is far more ubiquitous. The American Association for Artificial Intelligence (AAAI) recognizes and awards the inventors and developers of unique and particularly ingenious applications of AI. This year (2004), the AAAI awarded honors to applications ranging from software used to track and identify potential insider trading, to a program which schedules Norwegian train crews, to a robot designed to take spontaneous photos of participants at social occasions such as weddings.
A variety of intriguing philosophical questions have emerged over the past several decades concerning Artificial Intelligence. Can machines be truly intelligent? What is the nature of consciousness? Is artificial intelligence the same as "thinking"? Most scholars make a clear distinction between advanced, complex solutions possible in computers and the very human concepts of "consciousness" and "thinking" (McCarthy, 2003).
Whereas, the computing power of standard, desktop computers is expected to equal that of the human brain within the next couple of decades (Moarvec, 1998), computers are only as intelligent as the human input they receive. Computers are inherently rule-based, and humans must tell them what those rules are. It is unlikely that computers will ever will function with the same nuance and subtlety of human thought. However, Moravec (1998) noted that humans have peaks and valleys in cognitive performance. We're very good at complex actions such as language processing, visual recognition and social interaction. But, we're very poor at tasks such as rote memorization and large scale, rapid calculation. Computers are good at tasks that form the valley of human performance. He described the advancement of computer processing power as a flood that has already covered the valleys of human performance, but still has a long way to go to threaten the peaks.
These philosophical discussions are relevant and thought-provoking, but there is a pragmatic application for AI too -- creating machines that can make daily life easier, and allow a "hybrid" form of AI, one that interacts with humans and machines.
A recent report from Germany describes a computer enabled drill that monitors the hardness of the rock, the pressure applied by the human operator of the drill, the drill bit speed and contact pressure to adjust the motor speed to provide the fastest, most energy-efficient drilling through the rock (Frey et al., 2003). The system monitors both; the machine-based attributes, and the characteristics of the human operator to determine the optimal solution.
AI and Hearing Aids
Artificial Intelligence applies to advanced technology hearing aids too. Digital technology fulfilled one of the major expectations when it was released to the hearing aid marketplace in 1996: digital technology is indeed present in hearing aids across a broad price range.
Importantly, no longer is "digital" synonymous with "premium," it no longer defines the highest end of hearing aid technology. Rather, it has become the standard platform that nearly all new hearing aids use.
The key to advances in hearing aid performance will come from algorithm and software development. In other words, now that we have digital hardware, we need to maximize the capabilities of digital technology as it applies to human auditory perceptions, i.e., hearing!
This is where Artificial Intelligence comes in. AI is the vehicle through which new levels of patient benefit can be achieved in digital hearing aids.
The Nature of Everyday Environments
Think of the last restaurant you frequented on a Friday or Saturday night. From an acoustic perspective, what was it really like in there? Noisy? Reverberant? Was the waiter or waitress soft-spoken? Were multiple conversations going on? Were you seated near the hostess stand? Was the phone ringing and the front door opening every few moments? Was music playing? Was the television (or multiple tel;evisions) on? Now think of another restaurant you're familiar with, perhaps one you went to for a business lunch meeting recently. How different were these two environments? Probably day and night!
Communication environments and situations vary tremendously. Some situations are stable and predictable, while others aren't. Overall sound levels vary from situation to situation and moment to moment. Competing sounds vary, they come and go during conversations and even the location of competing sounds will change over time. Noise loudness levels, the "nature" of the noise, reverberation characteristics all vary, perhaps approximating an acoustic version of Brownian motion (for more on "Brownian motion"see web site reference, "Einstein Year").
How often are we in noise? What sort of signal-to-noise ratios are common?
A classic study by Pearsons and colleagues in 1977 (Pearson, Bennett & Fidell, 1977) provided an important corpus of data on typical speech and noise (S/N) ratios across a wide variety of everyday listening situations (see Figure 1). What is striking is that the average S/N ratio provides a significant challenge for most people with sensorineural hearing loss. Another important observation by Pearsons et al. (1977) was that as background noise levels increase, typical S/N ratio decreases! Talkers do not fully compensate for increased background noise competition.
Figure 1. Average speech and noise levels in a variety of environments, from Pearsons et al. (1977).
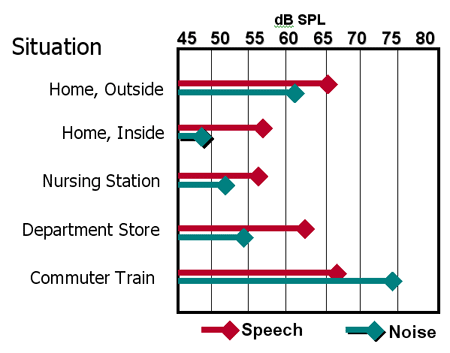
For example, when overall background noise level is 50 dB SPL, the average S/N ratio was approximately +7 dB. When the noise level increased to 70 dB SPL, typical S/N ratios were recorded at -2 dB. Therefore, as the acoustic environment worsens, the typical S/N ratio worsens, and the opportunity to clearly recognize speech decreases. Hearing impaired people, in general, will perceive sound best with S/N ratios of +14 to +30 dB. In other words, the signal needs to be significantly louder than the competing background noise (Staab and Lybarger) for listeners to perceive speech with maximal clarity.
Walden and his colleagues (Walden et al., 2003) recently reported on a classification scheme of typical communication situations encountered by hearing aid users. Their patients kept a diary of situations they found themselves in, noting how often they were in such situations and the amount of time spent there. The investigators classified the situations along a variety of dimensions (presence of background noise, presence of a primary talker, location of the background noise, amount of reverberation, etc.) They used the frequency of occurrence and duration information to calculate a metric of "total active listening time".
Based on this analysis, the two most common types of listening situations, accounting for one-third of the total active listening time, shared common characteristics; i.e., the primary talker was in front and close, with significant background noise present. The two most common situations varied only in terms of how much reverberation was present. The next two most common situations, accounting for another 25% of total active listening time, was also characterized by having a primary talker near and in front with low or high reverberation, but in these situations, background noise was not present.
Walden et al. (2003) reported that users of directional hearing aids prefer directional settings not anytime noise was present, but rather only in a more specifically defined set of conditions, such as when the talker is near and in front, and the noise arises from a location other than in front.
To improve performance in "noisy situations," noise management systems must deal with a complex set of variables, addressing issues beyond the absence or presence of noise.
Why Artificial Intelligence in Hearing Aids?
Given the complexity of real world communication situations, any simple classification of acoustic environments, such as "quiet" or "noisy" fails to capture the complex, relevant and ever-changing acoustic characteristics. As of this time, "prediction-only" approaches to signal processing have dominated hearing aid technology related to noise management. These approaches have been based on some measure of the input signal, to which an assumption was made, an algorithm was engaged and a prediction was made. Although this has been a reasonable approach and has served well in some domains (such as wide dynamic range compression), there are many communication situations in which uni-dimensional predictions cannot capture the true complexity of the environment.
Given the location of the talker in space as related to the hearing aid microphone(s), her voice loudness level, the location, level and spectral content of the noise in the room, the amount of reverberation in the room, one should query "Is a directional setting really the best at this moment in time?" What about when the user moves to the next environment? And the next?
A better technique is to confirm that the signal processing applied achieves the desired outcome.
A "confirmation approach" to the application of signal processing (described below) is a more direct solution, especially when the "prediction-only" approach does not achieve the desired result in every situation. Advanced technology hearing aid systems that perform sophisticated analysis of the communication situation and the acoustic environment, and adjust their performance based on whether or not specific performance goals are met, have a greater likelihood of meeting the needs of the user.
Upon reflecting on the importance of Artificial Intelligence in everyday life, Allen Newell wrote:
"Exactly what the computer provides is the ability not to be rigid and unthinking but, rather, to behave conditionally. That is what it means to apply knowledge to action: It means to let the action taken reflect knowledge of the situation, to be sometimes this way, sometimes that, as appropriate. . ."
Newell captured the very nature of why the use of Artificial Intelligence in hearing aids is so important. More traditional systems have not allowed us to meet all of the challenges faced by hearing impaired patients. In complex, challenging listening situations, our patients continue to seek more effective technology.
By moving into the era where hearing aid processing is properly described as an application of Artificial Intelligence, we offer a new, distinct advantage. AI provides a new way to communicate to the public about how advanced technology amplification is designed to operate.
Previously, we have told hearing impaired patients that new devices have been designed to handle noisy situations better and better. First there were noise switches, then multiple programs for quiet and noisy environments, then automatic low-frequency reduction, and then directionality. For patients wearing hearing aids for a number of years, they have seen a steady progression of new attempts to solve a longstanding problem.
The complex, decision making processes in Syncro allows us to use new terminology (AI) to describe new hearing aid circuit ability, and to re-invigorate interest in what hearing aids can provide for the wearer.
Artificial Intelligence in Oticon Syncro
Flynn (2004) described the core signal processing concepts of the Oticon Syncro. Syncro is an advanced technology, eight channel digital hearing instrument incorporating state-of-the-art implementations of adaptive directionality, noise management and wide dynamic range compression. Artificial Intelligence is implemented in the Voice Priority Processing (VPP) system which combines three signal processing approaches: Multi-band Adaptive Directionality, TriState Noise Management and Voice Aligned Compression. All of these systems are designed to work in progressive optimization of the signal with the focus on the speech. The unity of one single processing goal ensures that all systems are working in synergy and not in opposition.
Importantly, within the VPP system, processing is conducted in parallel and with progressive optimization of the signal. Parallel processing allows multiple solutions to be evaluated simultaneously to ensure the best solution is selected. For example, in four independent frequency bands, all possible polar plots are evaluated simultaneously to determine which one would provide the greatest reduction for sounds coming from the sides and rear. At the same time, three different directional mode options are evaluated to decide which option provides the best S/N ratio. Additional factors such as the presence of wind noise, the overall input level and the location of the primary talker are monitored and influence the selection of the optimal directional mode. Figure 2 summarizes the decision making structure in the Multi-band Adaptive Directionality system. Similarly, the amount of gain in each of the eight compression channels is primarily determined by calculations of the Voice Aligned Compression. However, these values will be modified by the decisions of the Tri-State Noise Management system. This system monitors the presence of speech in the environment. In parallel, it monitors the overall level and the S/N independently in each of eight channels. The information as to the presence or absence of speech, channel specific level and channel-specific S/N is combined to determine appropriate further modifications of the gain levels in each of the eight channels. Figure 3 summarizes the decision making process within the TriState Noise Management and Voice Aligned Compression systems. Compared to uni-dimensional, prediction-based approaches, in both of these examples, Syncro employs a multi-layered set of evaluation criteria, with confirmation that the chosen collection of settings meets the particular goal for that subsystem, with the overall goal of the system being that speech should be prioritized.
Figure 2. Flow diagram showing the variables which affect the decision of which directional mode (surround, split directional or full directional) is active in Oticon Syncro.
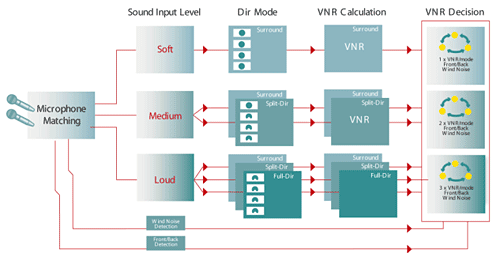
Figure 3. Flow diagram showing the variables which affect the gain level in each of the eight channels in Oticon Syncro
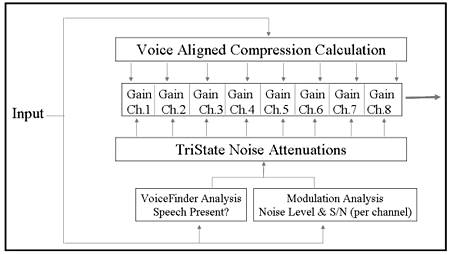
Syncro behaves intelligently in that at any given moment, it makes an accurate, multi-dimensional assessment of the sound environment and changes its amplification strategy to one which is optimal for the reception of speech in that particular environment. It detects whether or not noise is present, whether or not speech is present, from which direction speech is coming, what the overall sound level is and whether or not there is wind noise present. Synchro chooses the precise combination of directional, noise management and compression systems to provide the clearest possible speech signal for each acoustic environment. As the sound environment changes - Syncro updates its settings to optimize performance.
Rule-based Decision Making
There are two major approaches used with Artificial Intelligence applications (Champandard, 2003), they are the "Classical Approach" and the "Statistical Approach." The Classical Approach uses a predefined set of rules to analyze a set of input data to determine the best possible solution.
A good example of the Classical Approach would be air traffic control systems. A clear set of rules are defined: everything that goes up, must come down; no two planes can occupy the same space at the same time; planes can travel between 300 and 500 mph; it is desirable to arrive as quickly as possible. The rules can be hierarchical, with some rules absolute (what goes up must come down) whereas others are not necessarily absolute (shortest duration possible). The system can manage the take-off time, flight routes and air speeds of all flights in order to assure all planes arrive safely and, when possible, as timely as possible. Instructions to each flight are updated to reflect new information (e.g., storm systems requiring re-routing). Of course, safety is prioritized over timeliness.
A uni-dimensional approach could not handle these tasks with the efficiency or safety of the Classical Approach. If each plane were allowed to operate independently, traveling as quickly as possible to its destination, there would exist a high risk of danger. The Classical Approach is particularly useful in managing large data sets with multiple dependencies where the rules are clear and explicit. This approach is well-designed to handle changing and unpredictable date input (weather effects, take-off delays) while still using the rule-set to reach the desired solution.
An alternative technique is the Statistical Approach. In this approach, large sets of data are analyzed to find patterns and generalities. This is often referred to as machine learning and forms the basis of concepts such as "Fuzzy Logic" and "Neural Networks." The rules are not explicitly stated at the outset. Rather, rules are deduced by the system as an output of the data analysis.
For example, imagine a computer program designed to replace the celebrity judges on American Idol. The program might be given the task of predicting the next big superstar by analyzing a variety of input including; what sort of performers have already become superstars? What sort of music do they perform? What is the quality of their voices? How do they dress? How old are they? What hairstyles do they wear and, how attractive are they? The program would analyze the data to create the prototype of a superstar and would apply those criteria to choose the next performer likely to make it big. The Statistical Approach is designed to assist humans in finding patterns, rules and commonalities, that may not be readily apparent.
When viewing the design of an Artificial Intelligence based hearing aid, the Classical Approach is the appropriate choice. Like the air traffic control example, the rules can be explicit: find the polar plot that reduces the most noise, find the directional mode that provides the best S/N ratio, reduce gain more when speech is absent than when speech is present. The rules can be hierarchical: the detection of wind noise will override other rules that might call for full directionality. Most importantly, the system can handle unpredictable data: changing loudness levels, variable spectral content and changing location of noise, presence or absence of speech, etc. By analyzing many different potential solutions in parallel, the signal processing program in Syncro can apply the rule-set to unpredictable data input and determine the most satisfactory solution possible.
What about using the Statistical Approach? There are drawbacks to applying the Statistical Approach to hearing aid programming. In order for the program to deduce appropriate settings for new situations, the patient might need to hear many different potential solutions in a given listening environment and then decide if a given solution is desired, or not. Although theoretically possible, it is difficult to imagine a patient listening to and evaluating thousands of trials across different potential device settings in order to have enough data to develop a general solution.
The Classical Approach makes better sense, as we are able to set a series of reasonable rules and use these to manage device reaction to unpredictable environmental input.
Imitation vs. Understanding
Experts in Artificial Intelligence point out there is a difference in trying to create computer programs that imitate human brain physiology, versus those that try to understand basic challenges of the human brain while finding machine-based solutions to that problem (Ford & Hayes, 2002). Ford and Hayes drew a parallel with the Wright brothers ... Before the successful development of the airplane, earlier attempts at flight tried to imitate the way birds flew. These attempts universally failed. However, once the Wright Brothers set out to understand the basic principles of flight; speed, weight and lift, they created a machine solution consistent with their understanding of the principles of flight. Similarly, we understand the basic principles that govern speech understanding (optimal S/N, speech audibility, acceptable comfort) and have created an intelligent system to operate consistent with these principles.
Syncro is not designed to mimic the natural, human, auditory or cognitive systems in complex, dynamic listening situations. Rather, Syncro is designed based on our understanding of the complexity and variability of real communication situations and the signal processing approaches that provide the greatest benefit for patients with sensorineural hearing loss.
Conclusion: A New Mindset
The core benefit of using Artificial Intelligence in hearing aids is to handle the complexity of real situations, in real time, via rule-based, confirmed solutions - not just predictions in isolation. Applying AI to hearing aids allows new audiological solutions to be applied, through complex, problem-solving algorithms.
When digital technology was introduced to the hearing aid market place, it represented a dramatic shift in the core technology used in amplification. That watershed event was met with the expected range of reactions; from excitement and hopefulness to skepticism.
Over the past eight years, any legitimate discussion of the role of digital technology in amplification included some version of the statement: "It is not the technology itself that's important. It is what the technology allows us to do for the hearing impaired patient". That statement has never had more relevance than now.
We are defining the complexity of real sound environments in greater detail than ever before, and we are now seeing signal processing concepts that use complex decision making strategies to select the very best processing approach for any situation at any time. By introducing Artificial Intelligence into hearing aids, we can frame the professional discussion in terms of problems and solutions and not bits and bytes.
Importantly, we can re-energize or discussions with patients, their families and the public at large and show them how the focus on the development of advanced problem-solving algorithms has opened up new possibilities.
References and Recommended Web Sites
American Association for Artificial Intelligence (2003). IAAI-03 Summary of Applications. www.aaai.org/Awards/awards.html.
Champandard, A. (2004). Artificial intelligence plain and simple: Approaches. ai-depot.com.
Flynn, M. (2004). Maximizing the Voice-to-Noise ratio (VNR) via Voice Priority Processing. Hearing Journal, April: 54-59.
Ford, K & Hayes, P. (2002). On computational wings: Rethinking the goals of Artificial Intelligence. In S. Fritz (Ed.) Understanding Artificial Intelligence: 5-17. Warner Books, New York.
Frey, C., Jacubasch, A., Kuntze, H. & Plietsch, R. (2003). Smart neuro-fuzzy based control of rotary hammer drill. Proc. 2003 IEEE Int. Conf. .ICRA'2003, Sep. 14-19.
www.einsteinyear.org/facts/brownian_motion
www.ai-depot.com
McCarthy, J. (2003). What is Artificial Intelligence? www-formal.stanford.edu/jmc/whatisai/whatisai.html.
Moravec, H. (1998). When will computer hardware match the human brain? www.transhumanist.com/volume1/moravec.htm.
Newell, A. (1992). Fairy Tales. AI Magazine: 13 (4): 46-48.
Pearson, K., Bennett, R. & Fidell, S. (1977). Speech Levels in Various Environments. EPA-600/1-77-025. U.S Environmental Protection Agency.
Staab, W.J., Lybarger, S.F., "Characteristics and Use of Hearing Aids" in Katz, Handbook of Clinical Audiology, 4th Edition, Williams and Wilkens, Baltimore, Maryland. 1994.
Walden, B., Surr R., Cord, M. & Drylund, O. (2003). Predicting Hearing Aid Microphone Preference in Everyday Listening. Paper presented at the Annual Convention of the American Academy of Audiology, San Antonio.
Click here for more information about Oticon.