From the Desk of Gus Mueller
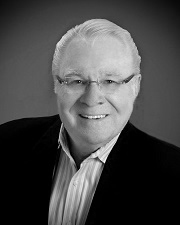
Many of us first started paying serious attention to artificial intelligence (AI) in 1997 when we learned that the world’s chess champion, Garry Kasparov, was going to play IBM’s computer, Deep Blue. in case you don’t remember, Deep Blue won. About the same time as this famous chess match, we also saw a few early reports illustrating how AI could be used in decision trees for differentiation of audiologic pathologies. That was over 20 years ago. Today we take AI for granted. It’s everywhere in our day-to-day activities: Google, Amazon, Siri, Alexa, Facebook, Netflix . . . and most importantly, AI can even be used to brew a beer to perfectly match our palate.
One area where AI, including machine learning, has made considerable advances in audiology relates to user-controlled, post-fitting adjustments of hearing aids. At least one manufacturer allows users to compare two different sound processing profiles for a given listening situation, and then select which one is best. The algorithm learns the patient’s preferences and presents other sound profiles that are similar, until an overall favorite is found. Moreover, the preferences for similar listening situations from thousands of patients are stored and sorted to fine tune the options provided for specific real-world loudness levels and SNRs.
Not surprisingly, in recent years AI also has been utilized in clinical vestibular assessments. Here to tell us about one approach where this has been successful is this month’s 20Q author, Devin McCaslin, PhD. Dr. McCaslin serves as the Director of the Vestibular and Balance Laboratory at Mayo Clinic in Rochester, Minnestota, and is an Associate Professor in the Mayo Clinic College of Medicine. He has authored numerous publications that cover the areas of tinnitus, dizziness, auditory function, and outcome measures development. His books, including on Electronystagmography and Videonystagmography from Plural Publishing, have become go-to references for both students and clinicians. He also serves as the Deputy Editor-in-Chief of the Journal of the American Academy of Audiology and is the Past President of the American Balance Society.
Devin’s major academic, clinical and research interests relate to clinical electrophysiology, vestibular assessment, healthcare economics, and the application of artificial intelligence to manage and treat dizzy patients. His excellent 20Q article gives us a glimpse of how artificial intelligence, machine learning and neural networks can be employed to assist us in our clinical activities.
Gus Mueller, PhD
Contributing Editor
Browse the complete collection of 20Q with Gus Mueller CEU articles at www.audiologyonline.com/20Q
20Q: Using Artificial Intelligence to Triage and Manage Patients with Dizziness - The Mayo Clinic Experience
Learning Outcomes
After reading this article, professionals will be able to:
- Discuss what is meant by the term artificial intelligence and list a potential application in audiology
- Describe the difference between machine learning and deep learning
- Explain how AI is being used in a vestibular clinic

1. Everywhere I look there are reports that artificial intelligence is being used to solve problems in healthcare. Is it everything they say it is?
It all depends on who you ask. First, let’s break down what we mean by “artificial intelligence” or AI. When we think of AI we often connect it to what we see in the movies. For example, The Hitchhikers Guide to the Galaxy, War Games, Terminator, and Star Wars all conceptualize AI as something that is capable of thinking and acting like humans. Although this is an exciting idea about what AI can be, the technology is really in its infancy. AI means many different things to different people depending on the application. The word artificial would indicate that humans are not doing the operations being performed. Intelligence, however, is a much more difficult term to define. At this time there are no computers that can even come close to performing mental functions as a human can. Even given that fact, there is tremendous application for the use of AI in the healthcare space.
2. Ok, well that is somewhat disappointing. What is AI currently capable of doing?
Basically, AI refers to using computers to solve problems on their own. Many different types of AI are being used in our day-to-day lives that we don’t even notice because AI is so efficient. For example, personal assistants like Alexa, the mapping system on your phone, and your online music system all incorporate AI. Additional applications using AI include banking and scheduling your flight. But most exciting to me, is how AI is being used to help our patients. The reason for the AI hype, and I believe it is well-founded, is that it offers numerous advantages over traditional clinical decision-making techniques and traditional data analysis.
3. Is this technology available to help those of us that work with patients with hearing impairment or dizziness?
The short answer is yes. The healthcare industry and computing technology are finally both at a point where the enormous amounts of complex patient data can be assimilated and evaluated in a meaningful way. With the transition to the electronic medical record over the past 10 years, patient health data are now able to be tracked over the patient’s lifetime and extracted and analyzed with millions of other patients. This has enormous implications for those of us that work with people with hearing impairments and patients with dizziness. One exciting example is the new generation of hearing aids that incorporate AI techniques to assist patients in hearing more clearly in different environments. There was also a paper recently published entitled “The Use of Artificial Intelligence to Program Cochlear Implants” by Waltzman and Kelsall (2020). We are beginning to see this type of technology more and more in our profession.
4. I know you work with patients who have dizziness. Can we use AI to help with their diagnosis and treatment?
Absolutely. In fact, we are using AI techniques now to help us better manage and treat our patients. Dizziness is challenging because there can be many reasons why a patient is dizzy. In some instances, the dizziness may be due to an inner ear or balance problem and an otolaryngologist, audiologist, or physical therapist is the best healthcare provider to treat them. Other patients may have dizziness that is best addressed by a neurologist or even a psychiatrist. Over the last three years our team has been developing a system that uses a form of AI to automatically triage patients with dizziness to the clinician who can best treat their condition.
5. That sounds extremely helpful for both the patient and the clinician. How does it work?
Well, first we need data for the system to analyze so that it can provide us with its best guess regarding who is most likely going to have the best chance of treating the patient’s dizziness. We collect the data using an online questionnaire that the patient fills out. The questionnaire covers areas such as symptoms, family history, medical history and previous tests they have undergone. The patient can enter the data from a computer anywhere or even on their mobile phone.
6. It sounds like you are just doing what we have been doing with pencil and paper for years. Is there a difference?
Good question and you're right, it is similar. In our case, the data needs to be in what’s called a structured form so that the system knows where to find certain features or patient responses that are important for helping it make predictions. We refer to this as predictive modeling. An example of a structured database that is similar is when we enter data into Microsoft Excel in columns. The power of this approach is that all the patients’ data is in the same place. This allows the system to analyze all the patients’ responses and look for patterns. We currently have approximately 2000 patients in our database. Since each questionnaire is 162 questions, we currently have almost 324,000 pieces of data. For dizziness, certain patterns of symptoms are associated with particular disorders like Meniere’s disease, vestibular migraine, and benign paroxysmal positional vertigo, to name a few. Building a system that is capable of processing all these data at the same time to solve a problem and not be overwhelmed is often referred to as a “big data” approach.
7. Okay, that’s a lot of data, but where does AI come in?
You are correct, just having a lot of data in a database is not AI. You have to have something that can analyze all that data. The analysis of the data is performed, in our case, by an algorithm. An algorithm is a mathematical sequence that generates a solution based on an analysis of a set of data. It may also tell you that there is no solution to the problem it is tasked with solving. An AI algorithm is trained to solve a problem that was typically done using human intelligence. As humans, we use algorithms all the time in everyday life. For example, in the case of a conductive hearing loss the audiologist might identify that a patient has better pure-tone thresholds for bone conduction than for air conduction, a flat tympanogram and absent acoustic reflexes. The audiologist then categorizes that as a conductive hearing loss. If the data from the audiogram is in a structured format, a computer could be trained to detect that constellation of findings. In fact, this is already happening in auditory diagnostics. So, the AI part is that humans can train an algorithm to perform a function they do.
8. What problems do the dizziness algorithm you mentioned solve?
Well, when I arrived at Mayo Clinic in Rochester, Neil Shepard had already assembled a multidisciplinary clinic to assess and manage patients with dizziness. This team is referred to as the Mayo Integrated Neuro-vestibular Team or MINT. The MINT is a clinic that is composed of different subspecialists with expertise in dizziness. As I mentioned before, the challenge was to teach a computer to identify which specialists were best suited to treat the patients and avoid scheduling patients with those that would have nothing to offer. We have developed an algorithm that can automatically triage dizzy patients to the physicians that can best treat their form of dizziness.
9. How did the idea of using AI to solve this problem arise?
It was self-preservation and the need to see patients in a more timely fashion that drove us to find a solution. Having our team manually analyze all of the incoming patient questionnaires and work through volumes of external records (that were often not helpful) took an enormous amount of manual effort and time. That effort and time could instead be allocated to seeing patients. In fact, we have referred to this project as the “Martini Project”. The idea was hatched in a Martini bar in Rochester, Minnesota. Neil and I went out one day after work and I pitched the idea to him about developing an algorithm to manage our triage program. From there, it became a reality.
10. Can you tell me a little more about how algorithms use the data to make decisions? For example, a recipe in a cookbook is an algorithm but that's not AI.
Good point. An algorithm is simply a procedure that follows a set of mathematical instructions. In our case, we had to tell (i.e., program) the computer what responses from the patients' questionnaires were associated with which disorders. Then, we had to teach the computer to pick the providers that were most appropriate to treat the particular disorder/s. The core difference between a simple algorithm and one that incorporates AI is that the AI algorithm can modify itself based on incoming data to make informed decisions. This is sometimes referred to as a “learning algorithm”. Learning algorithms become more efficient and accurate as they gather more data and feedback. In our case, the feedback is an outcome measure known as the Dizziness Handicap Inventory, a self-assessment scale developed by Gary Jacobson and Craig Newman in 1990. Over time, our system will begin to adapt its performance and decisions based on how patients report they are doing following treatment. The ability of the algorithm to adapt rather than just operate when certain triggers are encountered is what the term “intelligence” refers to in AI. One common type of AI algorithm you may have seen referenced is referred to as machine learning.
11. Yes, I have seen the term machine learning. I also see the term deep learning in reference to AI. What’s the difference?
Mathematical models that have the capability of learning or modifying their solution from incoming data fall under the broad heading of “machine learning”. In short, machine learning refers to a set of AI procedures that become more accurate at discovering patterns over time. With a machine learning model, and it is true with the one we currently use for dizziness, humans supervise the predictions and will adjust it when erroneous decisions are detected. For our system, we review the final AI chosen schedule of appointments and determine if we agree. In most instances, the model performs well - but not always. Deep learning is a type of machine learning where the algorithm determines on its own if the prediction provided was correct or not. With deep learning systems, there are often more than one algorithm working together. This is often referred to as a “neural network” which is used when there are extremely complex problems to be solved.
12. If I wanted to make an algorithm what are the steps? I would love to have one that would suggest appetizers based on what I am having for dinner.
This was the fun part of the project. The first step is to clearly define and outline the task you are interested in having the algorithm solve for you. The second step is to gather the data that will be used to train the algorithm. Once you have the data it must be organized in a randomized form so you don’t have any bias. Next, the type of model that will be used must be decided on and different models lend themselves to certain tasks better than others. Picking the correct type of model usually requires an individual with some background knowledge in predictive modeling and AI development. This will save you a lot of time. One book I have found helpful is entitled Applied Predictive Modeling (Kuhn & Johnson, 2016). Once you have decided on a type of model and you have the training data-set, you are ready to train the model.
13. What’s involved in training an AI model?
Training is pretty simple. It requires running your data through the system and continually adjusting it to obtain the correct answer. For example, in our case, the goal for our model was to use patient symptom data to choose the best clinician to treat their problem. In order to do this, we randomly selected a large number of medical records from patients that had completed our program. These patients had filled out our patient questionnaire so we knew with great detail about their presenting symptoms. We also knew what their diagnoses were since they had been treated by our team. Our multidisciplinary team worked together to agree on what the most appropriate appointments were for each case. Specifically, not what appointments the patient had had attended originally, but rather, what appointments should they have had based on the diagnoses. We were then able to determine statistically, which symptoms were most associated with which diagnosis and subsequently who would be the best medical professional to treat them.
14. Did it work?
In order to determine how successful or accurate the model's predictions are, the model must go through what is called a validation phase. Our team randomly selected another set of retrospective patient data that is referred to as validation data. The multidisciplinary team compared the model's output using this new set of data compared to the team's consensus decision on the best set of appointments. From that comparison, we could identify where adjustments needed to be made. The model was adjusted and we were ready to test it with new patients.
15. When you tested the revised algorithm with new patients coming to your clinic, what was the outcome?
It performed better than expected. In order to evaluate its success, our hospital assigned an independent reviewer to assess its performance. The model's performance was evaluated based on a number of metrics. Three important metrics were the algorithm's accuracy, savings to clinician’s time as compared to manual triaging, and average number of appointments saved.
16. What was one of the surprising outcomes using this type of technology in your practice?
I'd have to say it was our initial finding of an approximately 20% drop in dizziness referrals to otolaryngology. This was pretty surprising. Many healthcare professionals that see patients with dizziness automatically refer them to an otolaryngologist. We found that in our system, there was a significant over-referral to our ENT colleagues. The algorithm rerouted these patients to different subspecialties that may have more to offer for their condition.
17. Is your system learning and adjusting itself based on the patients' outcomes?
Not quite yet. I learned that the system needs data to begin to make good decisions. We have moved from “heavily supervising” the model's output to “lightly supervising” the model's output. Supervising refers to monitoring the model's prediction to ensure it is making good decisions. When we disagree with one of the appointments it has chosen we change it and the model registers the change. Once we have enough data in the system we will let it begin to make decisions and to modify itself by using the patient outcome measures.
18. Once you have this operating effectively, is it something that could be used by other clinics?
Our goal from the outset of the project was to develop an intelligent system that would be capable of providing clinicians anywhere in the world with a new tool capable of guiding the treatment and management of patients with dizziness. Although it has surpassed all expectations at Mayo Clinic, the challenge was to adapt the system to operate effectively in other environments such as private practice and governmental healthcare organizations. To accomplish this task, we have partnered with a Danish healthcare company called inno.health. Our industry partner has assembled an international advisory board to guide the process of further developing the Mayo Clinic algorithm to adapt to the varied types of healthcare systems that exist globally as well as help with distribution.
19. What are the next steps?
We have a number of projects in the works that utilize the algorithm. One question we are very interested in is determining how we can incorporate vestibular laboratory testing into the model's predictions. We are also beginning to work towards analyzing the relationships between patients' diagnoses with treatments and patient outcomes. These are some of the areas that are really exciting for us.
20. Is AI is going to replace those of us audiologists that are seeing patients with dizziness?
It’s a great question. I don’t see that happening. What I do see happening is AI acting as a decision support tool to assist with recommending appointments and treatments. When we set out to build the system we wanted to accomplish three things. First, we wanted the achieve the fastest time to the correct diagnosis. Secondly, we wanted to obtain the diagnosis with the least amount of cost to the healthcare system. Lastly, we wanted to achieve the best outcomes for the patient. I think we are on the right track and we are already learning a lot from this approach. For those of us seeing individuals with dizziness, AI is already proving to be a useful tool in our management of these patients.
References
Jacobson, G.P., & Newman, C.W. (1990). The development of the Dizziness Handicap Inventory (DHI). Arch Otolaryngol Head Neck Surg, 116, 424–427.
Kuhn, M., & Johnson, K. (2016). Applied predictive modeling. New York: Springer.
Waltzman, S.B., & Kelsall, D.C. (2020). The use of artificial intelligence to program cochlear implants. Otology & Neurotology, 41(4), 452-457.
Citation
McCaslin, D. (2020). 20Q: Using artificial intelligence to triage and manage patients with dizziness - The Mayo Clinic experience. AudiologyOnline, Article 26880. Retrieved from www.audiologyonline.com